Weekly writing about how technology and people intersect. By day, I’m building Daybreak to partner with early-stage founders. By night, I’m writing Digital Native about market trends and startup opportunities.
If you haven’t subscribed, join 70,000 weekly readers by subscribing here:
Models Aren't Moats: Three Signals Amongst the AI Noise
The market feels a lot like 2021 right now. Things are frothy.
From seed to growth, AI deals are getting done at nosebleed prices and sky-high multiples. It’s interesting to see this happen when many tech companies are hurting from raising at inflated prices just a few years back. We just saw this movie—like, yesterday.
Say you raised in 2021 at 100x ARR. You would have to grow 100% a year for 5-6 years just to grow into that valuation (because of multiple compression). That’s to say nothing of raising at a 2x, 3x step-up. A lot of companies today are quietly raising down rounds or shutting down altogether. Many—maybe most?—unicorns will never be valued as highly as they were in 2021. Same goes for VC firms: many firms that lost discipline in the post-pandemic run-up are struggling to raise subsequent funds, and will quietly shutter.
It’s a self-serving VC talking point, but raising at too high of a price ends up burning everyone: founders, investors, employees. My former colleague Mark summed it up well in this 2022 tweet:
You might be able to repeat Mark’s tweet in a decade for Safe SuperIntelligence ($32B, $0 revenue), Thinking Machines Lab ($10B valuation, $0 revenue), or Cohere ($5.5B valuation, reportedly ~$20M ARR at the time).
Companies with just a few million in revenue (or no revenue!) are raising at nosebleed prices. If they end up being the next Google or Datadog or Wiz, great. But most won’t, and they’re going to struggle to raise flat or up rounds in a few years. The above names might not be great examples—they’re led by strong ‘n of 1’ founders—but many AI companies will feel the pain in a few years.
For multi-stage firms, this is the game: they need to be in one of the generational companies being built. Their model (and brand) is predicated on it. My friend Eric put it well this week:
And these firms often have to place multiple, conflicting bets to hedge their bets. My good friend and former colleague Kyle, now Eric’s colleague, put out this spicy tweet this week:
Something I’ve also been thinking a lot about: the quality of revenue that AI companies are being valued on.
I liked OnlyCFO’s provocative piece Yes, ARR Is Dead, which unpacks how low-quality some of this AI revenue is. Annual Recurring Revenue is a beautiful thing because it’s recurring—it’s right there in the name!—and because it’s predictable, high-margin, and typically expands as customer adoption broadens. In the world of AI, though, much of the revenue is experimental. It’s not really recurring; it’s not really predictable; it’s lower-margin; and churn is way worse. So should we really be valuing companies on the same ARR multiples?
I’ve been thinking about what signals we can glom onto amongst all this noise. I’ve arrived at three, which are the focus of this week’s piece:
Talent
Brand
Feedback Loops
Let’s touch on each.
Talent
One of my favorite sayings in tech is, “Every problem is a people problem.” I think this is originally a Patrick Collison saying from Stripe, but you now hear it often in the Valley. And it’s true: virtually every problem can be solved with the right talent.
In a frothy market, a good rule of thumb is: follow the talent. Why does talent matter?
As a founder, about half your time will be spent on people-related stuff. The composition of that time changes, but the percentage stays consistent at ~50%. In the early days, you’re sending cold outbound messages on LinkedIn and convincing people to join your fledgling company. Down the road, you’re managing execs and aligning your leadership team.
My old firm, Index, compiled this data in the Scaling Through Chaos book (worth a read). Here’s how founder time breaks down:
That’s a lot of “people stuff.” Of course, this also matters for non-founders. If you’re looking to join a startup, it helps to know (1) which to join, and (2) when to join.
Startups are scaling faster than ever: the time to 1,000 headcount compressed by ~30% over the course of the 2010s. AI is making people more efficient, making it possible to do more with less (this was a topic in the most recent 10 Charts series), but I still think the headcount trend will continue. With more venture dollars pouring into startups, and the “size of the prize” in tech ever-growing, more companies will be scaling their teams and competing for top talent.
One popular tactic for hiring in startups (and one that I suggest to founders): write down the five most impressive people you know. Then go hire them. This was the tactic that Facebook used in its early days; Keith Rabois talks about it in his talk How to Hire. This tactic allows you to build networks of top 1% people. And top 1% tend to hang around other top 1% people, so the networks grow stronger over time.
My former colleague Mark, who posted the tweet above, likes to use the term “Talent Vortex.” This is when a company builds an incredibly dense talent base, pulling A-players from other top companies.
An example is Persona, a company I worked with at Index, which drew top talent from other talent vortexes—primarily Dropbox and Square, where the Persona founders came from—to build a fleet of top 1% engineers, designers, and product thinkers.
Persona, like many top companies scaling quickly, would track where talent was coming from. The founders noticed compounding advantages: if you work at Square and you see one of the company’s top engineers post on LinkedIn that he’s joined Persona, you might start looking into a job at Persona. Talent recognizes talent, and good talent likes to follow good talent. When you start hiring one A-player, more are almost certain to follow.
Talent vortexes also often become the best pools for founder talent. The new ‘PayPal Mafia’ is the ‘Palantir Mafia,’ and so on. (Palantir alums have founded companies as wide-ranging as Anduril, ElevenLabs, and Partiful.)
For startup hiring, I’m a proponent of hiring the young, hungry, inexperienced but high-slope person. I asked my former colleague Charlotte Howard at Index her best advice for founders on hiring:
Don’t limit yourself to cookie-cutter profiles. The best hires aren’t always the obvious ones. Look beyond experience to core abilities—curiosity, adaptability, urgency, and resilience often matter more than having “done it before.”
Slope over intercept.
Charlotte also suggests dedicating real time to meeting with talent. Most founders will say that talent is their top priority, but calendar auditing reveals something else. If hiring and nurturing talent is #1, why are you only spending three hours a month on it?
Pear’s Matt Birnbaum, who has built out a robust talent function at Pear, says something similar. In response to my question on what founders should prioritize, he told me:
Many founders think that as their companies scale, they will become less involved in hiring. While their day-to-day involvement in hiring may shift (spending less time on top of funnel and more bottom of the funnel activities) the total time they spend hiring will never decrease (unless your company is failing). So you better learn to like it and, more importantly, learn to be great at it.
Match the calendar to the priorities.
Also from Matt:
Founders underestimated the importance of the first hire they make on their hiring bar. You’re setting the tone for the quality of all future hires. It’s incredibly difficult to reset the bar once you've made your first handful of hires.
This gets at one of my other favorite sayings: A-players hire A-players, and B-players hire C-players. This saying is cliche, but it’s also very true. If you let the talent bar slip, you’ll likely never get it back.
Brand
Brand is a controversial moat: it’s “squishy” and understandably less definable / tangible than other moats. But I’ve always been a believer that brand is a moat, and this is especially true in the age of AI.
Brand is more than a logo, typeface, and color palette; it’s about how your customers talk about you. It’s a narrative. Many AI companies have mastered brand. The most obvious is OpenAI with ChatGPT, which has rapidly become a household name.
One question I ask myself (for consumer companies): will my aunt in Ohio know this brand? In startup world, we live in a bubble of early adopters. But will your average American know this company and love its product? My aunt might not know OpenAI, but she knows ChatGPT.
She definitely doesn’t know Perplexity. With companies like Perplexity, I worry that they’ll skim off the early-adopter user base, but then plateau. Google is a mighty competitor; it’s brand is, you know, pretty good. ChatGPT has an early mover advantage in chat as an alternative to Google Search, but I’m seeing my non-tech friends finding Google’s AI Overviews more than good enough.
Good brands capture attention that far outweighs their traction—and they then use that attention to manifest traction and ultimately category-leadership. Runway is a good example. Is Runway really the best product in AI video? I would say no. But Runway has been incredibly savvy at brand-building. The company boasts 244K Twitter followers and is consistently demo’ing new features and inserting itself into the zeitgeist.
Contrast this with Kling, which only has 82K Twitter followers and doesn’t seem like it’s quite cemented itself as synonymous with the ‘AI creative tooling’ space in the same way that Runway has.
Sam Altman is also consistently savvy at building the OpenAI brand. Tweets like this continue to cultivate the OpenAI mystique + aura:
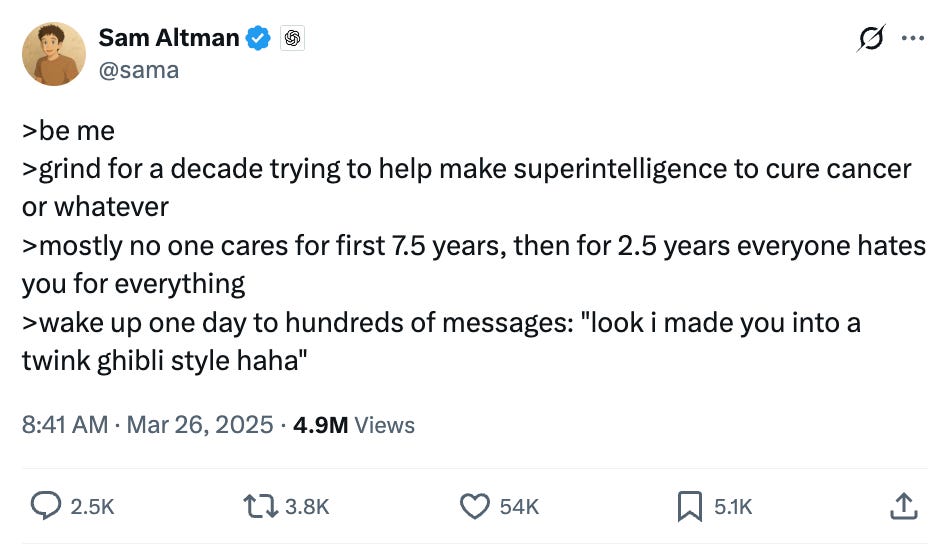
Brand can come from a lot of things. Ultimately, it derives from a great product. But it also comes from good design (you will always find me screaming from a mountaintop, “Hire a designer earlier than you think!”), from good storytelling, and from the ‘aura’ that comes from talent density (see: talent vortexes above).
It’s hard to define a good brand, but you can tell when a company has it.
Feedback Loops
Konstantine Buhler recently wrote a piece called Usage Is the Moat about why AI usage data is so critical. I like that framing. When everyone has access to the same foundation models, differentiation comes from how you learn and adapt your product.
This is the other advantage to look for in a crowded, frothy market: do you have a data advantage?
The challenge here is that this is where the rich get richer:
Notion’s flywheel comes not from the underlying model, but from the usage data—Notion understands how millions of users are organizing, summarizing, and editing content. This informs feature prioritization and keeps Notion ahead of competing tools.
Every time a developer uses Ghostwriter, Replit sees how code suggestions are accepted, edited, or ignored. That feedback loop, which is embedded in workflows, helps Replit fine-tune and improve over time.
Character.ai gets a treasure trove of data on the billions of messages that its millions of users are sending and receiving. Every conversation sharpens tone, pacing, emotional resonance.
The other important thing to note: not all data is created equal. We want contextual, user-specific, product-relevant data. Jake Saper from Emergence had an interesting post on LinkedIn this week. From the post:
I had dinner the other night with a fascinating group of folks building AI-enabled products. PMs from companies like Perplexity and Harvey, plus engineers and founders. One question kept coming up:
Why haven’t incumbent software providers been able to leverage their valuable data assets yet?
Both giants like Salesforce and growth-stage companies with 10+ years of collected data seem to be struggling to deliver truly differentiated AI experiences. Most of us would assume these companies are sitting on data gold mines that should give them a massive advantage. But is that actually true?
One perspective I found compelling: The historical data these companies have collected simply isn't the right kind to make AI models truly effective. The workflow data, outcomes data, or database info they've aggregated over the years might not be as useful as we'd think. What's most valuable in building agentic AI isn't just workflow data or outcomes data—it's a granular understanding of how humans actually perform tasks.
The counter perspective that Jake offers is that incumbents aren’t failing, but are just slower. Their data may, in fact, be excellent—and the products they release in the next 12-18 months will leverage that data to outmaneuver AI upstarts. Time will tell.
The point is: feedback loops are the silent moat.
Other moats are louder, but it’s hard to compete with high-quantity and high-quality data. You can copy UI and you can rent compute, but you can’t easily replicate a fast, tight, proprietary loop trained on millions of live, structured, product-relevant interactions. As those loops tighten, the product gets smarter, the experience gets smoother, and the gap between you and the next best alternative quietly compounds.
Final Thoughts
Thanks for bearing with me on some wide-ranging musings this week. These are all topics that have been on my mind. When the market is giving you a lot of noise, look for the few signals that are consistently reliable.
We’ll end on an image that I generated in Midjourney for the cover art for this piece. My prompt was asking Midjourney for a cartoonish image of knights using a moat to guard their castle. The irony here is that many people think that ChatGPT’s new image model has killed Midjourney. Does Midjourney have a moat? The good news for Midjourney is that they have the talent density; they have a strong, well-defined brand; and they have early data feedback loops that will compound over time. They’ll be just fine.
Thanks for reading! Subscribe here to receive Digital Native in your inbox each week: